ChatGPT in a World of Digital Trust
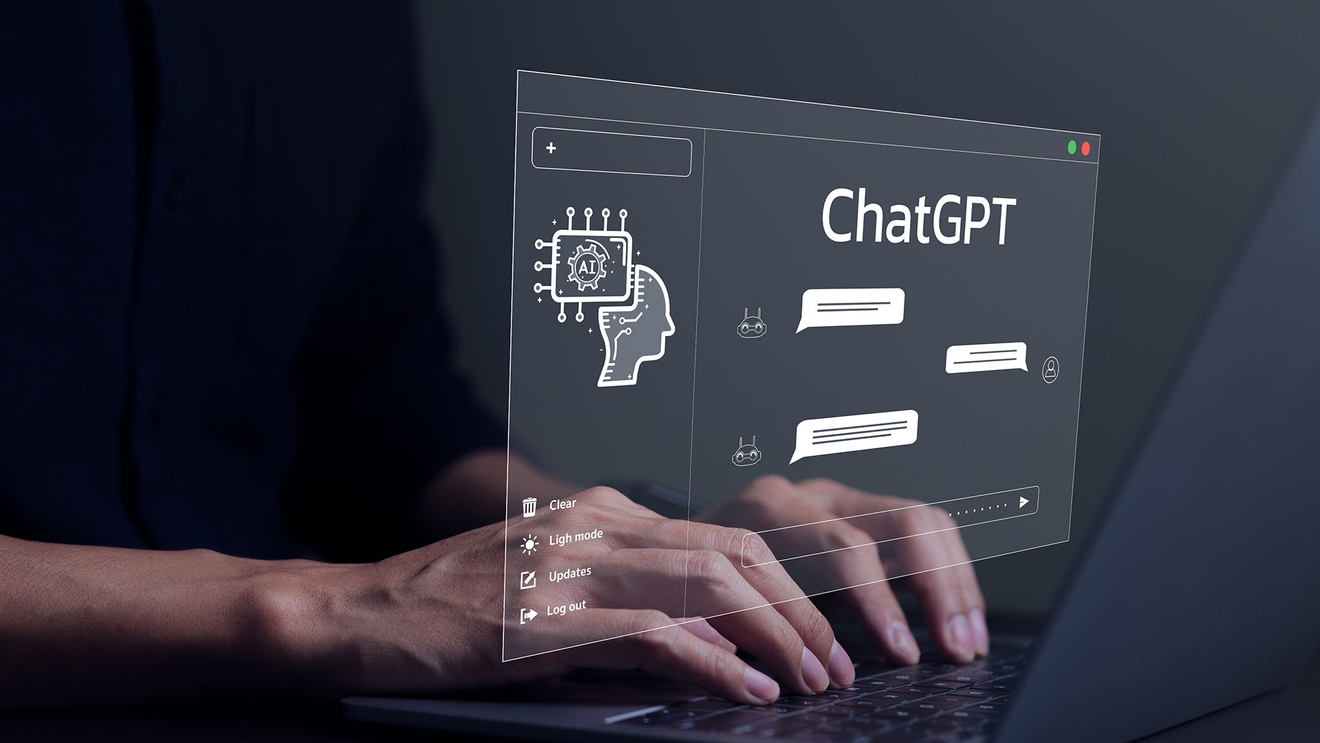
ChatGPT has received a lot of attention for its sophistication, speed to market, and impressive customer use rate. According to reports, it achieved 100 million active users within two months after launch, which is a record. But ChatGPT, a chatbot that can generate natural language responses to almost any question or topic, is only one of several use cases of artificial intelligence. There is a lot more you should know about this technology.
What is it?
The “GPT” in ChatGPT stands for generative pre-trained transformer. Generative AI is a type of artificial intelligence where computer algorithms can produce new content like something humans would create. These outputs can be text, graphics, images, audio, video, music, and even computer code. ChatGPT and DALL-E, both products of the company OpenAI, are good examples of this technology based on machine learning processes called neural networks that resemble the human brain.
This type of AI learns by analyzing the structures, relationships, and patterns of large amounts of training data used as examples of the desired output to understand the rules governing the content. Through fine-tuning, the model mimics, improves, and generates sophisticated and convincing content that shares characteristics of the training data. The results appear increasingly authentic as opportunities for refinement are identified and fed back to the models for further training creating a cycle of constant improvement.
Large language models (LLMs) are a form of generative AI that emerged around 2018 and consist of a language model trained on data sets several trillion words in size. By scaling the sophistication of the model, the size of the training data, the cost of training, ease of the human interface, and computing power for fast performance, these LLMs can be used for a variety of tasks. They can predict the next word or sentence and after deciphering the syntax and semantics of human language, have been proven very successful as chatbots.
OpenAI’s ChatGPT rapid growth has captured the attention of other companies like Google, Microsoft, and Amazon, who have launched generative AI LLM products of their own.
Types of Models
There are different types of models:
- Transformer-based models are trained on large amounts of data to learn the relationships within it, like sentences, so these are useful for generating and performing text-related tasks. Some examples are the real-time translation of text and speech, sentiment analysis, and object recognition. Auditors can also use these models to detect trends and anomalies to identify potential fraud.
- Generative Adversarial Networks (GANs) consist of two neural networks that compete against each other. One network is a generator that creates content, and the other is a discriminator that learns to differentiate and evaluates the results. Through an iterative mechanism where the two models compete against each other, both networks improve and produce more realistic results. Some examples include the generation of images, transformation of text to images, face aging, and video creation.
- Variational Autoencoders (VAEs): These models use an encoder to input data and simplify it, and a decoder takes the data and restructures it to resemble the original input data. These models have been used to separate source data like music and speech, image processing, detect diseases using electrocardiograms (ECG) signals, and options trading in finance.
- Multimodal models process multiple types of data, like text, images, and audio, and combine them to generate more sophisticated outputs. Examples include DALL-E 2 and ChatGPT-4.
Benefits and Use Cases The most common benefit of generative AI is efficiency as workers can automate tasks and focus on other activities. In addition to the examples mentioned above, case uses also include:
- Writing and checking computer code
- Generating new ideas and designs
- Creating marketing content
- Writing essays, poems, songs, articles, and other types of text
- Enabling chatbots and virtual assistants to improve customer service
- Analyzing data during research and for decision making
- Creating music, video, and images
Digital Trust
Digital trust is the confidence that people have in the digital systems and platforms they use daily. Users expect technology and related processes to help create a secure digital world where security, reliability, privacy, and the related data are used ethically across platforms and devices. It divides services that are dependable and instill confidence from those that are unreliable.
Digital trust is essential to building relationships between users and organizations, and making sure those interactions and the related transactions are handled according to expectations. This way, the parties involved believe they are safely getting what they are paying for.
Online commerce and personal communications depend on users trusting the organizations that are collecting, processing, transmitting, and storing that information. They expect the information to be handled securely and responsibly. Without digital trust, users would not engage online with confidence. Surprisingly, however, digital trust is too often assumed and receives inadequate attention and funding, even though it is a key component for the success of many organization’s business models.
Digital trust, then, becomes essential for organizations to fully benefit from the deployment of the many technological advances we are witnessing; but there are potential pitfalls.
Risks, Controls and Governance
Generative AI has no conscience and learns from the data it was fed, so bias and unethical concerns exist. Instances of lending apps denying loans, HR recruiting bots skipping job applicants, and the creation of deep fake images, audio, and video that can spread misinformation have been the source of concern.
In 2023 OpenAI confirmed that a glitch caused it to leak the conversation histories of some of its users to others. The organization archives and displays past conversations so users can keep track of their text inputs with the program. Although the organization indicated this was a mistake and not a hack, it raised concerns among its users and large language model critics.
The misuse or infringement of intellectual property has also gained a lot of attention since training data may consist of the content from millions of websites, audio files, images, and video materials. Content creators and owners worry they are not being appropriately compensated for the use of these assets. In a world where workers and the population at large increasingly rely on technology for business, education, and personal use, the following scenarios warrant attention and underscore the importance of digital trust:
- Cybersecurity threats: They can compromise the confidentiality, integrity, and availability of systems and data. The results can be reputational damage, financial loss, operational disruptions, and legal liabilities.
- Digital divide: This can increase the gap between those individuals that can benefit from digital technologies and those that do not. The result can be workplace and educational inequalities.
- Data privacy compromise: This can occur during the collection, use, sharing, and storage of sensitive information without the consent of the owner. The result can be the loss of trust and subsequent customer abandonment, public backlash, and legal liability.
In terms of regulations, the EU proposed new copyright and transparency rules in April 2023 that would require organizations to disclose if they used copyrighted materials. This proposal has been welcomed by many because the widespread use of online data as training materials for generative AI have often included contents scraped from millions of online resources, yet it remains almost impossible to identify, and scrutinize the specific sources used to train these models. In terms of output, newer releases of OpenAI’s ChatGPT, Microsoft’s Bing, Google’s Bard and other chatbots have begun showing some of their sources, but more transparency has been called for.
The General Data Protection Regulation (GDPR) enacted in 2016 in the European Union has become the model many countries and US states have used to design their own data protection and privacy regulations. It helps to enhance the control and the rights individuals have over their personal data, and a baseline to build upon at the national and corporate levels.
These dynamics reveal that low levels of digital trust can cause a host of negative outcomes including reputational damage, privacy breaches, cybersecurity incidents, loss of customers, lower reliability on the data for decision-making, lower revenue, and a decrease in innovation; all outcomes we wish to avoid.
Looking Ahead
Throughout history, technology and innovation have led, while controls and regulations follow. As such, auditors should continue to encourage their clients to put in place policies and practices that encourage digital trust and compliance with existing, and evolving, frameworks and regulations.
Digital trust is essential for cybersecurity and for the continuing success of our digital ecosystem, yet ISACA’s State of Digital Trust report indicates that few organizations have sufficient staff dedicated to digital trust. This is a cause for concern and an opportunity for auditors, regulators, and other professionals to gain relevant skills, align digital trust with organizational priorities, encourage leadership buy-in, seek additional funding for training, and to acquire technological resources.
We are witnessing the use of generative AI to accelerate the discovery of new drugs, personalize marketing campaigns, create educational materials, generate music and video content, predict economic movements and perform financial analysis, improve the quality of search engine results, and predict weather patterns. The number of use cases are expected to continue to grow as the technology is tested, and humans find innovative ways to use it. We should always remember that the rise in the number of its applications also requires an increase in our understanding of its implications.
By taking the lead and promoting digital trust within our organizations, we can collectively increase the level of digital trust and safely enjoy the benefits that these models are producing.
Further Reading:
Generative AI defined: How it works, benefits and dangers (techrepublic.com)
What Is a Transformer Model? | NVIDIA Blogs
OpenAI Confirms Leak of ChatGPT Conversation Histories | PCMag
18 Impressive Applications of Generative Adversarial Networks (GANs) - MachineLearningMastery.com
State of Digital Trust | ISACA
Dr. Hernan Murdock, CIA, CRMA is VP — Audit Content at ACI Learning where he supports the development of the organization's learning and development business, and the implementation of strategic initiatives to expand into new subjects, markets, and course delivery modalities. An author, instructor, and audit practitioner, Dr. Murdock has helped clients achieve their governance, risk, compliance, and operational goals for over 25 years across multiple industries in the Americas, Europe, Africa, and Asia. Contact Hernan